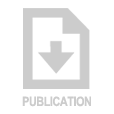
Citation:
Arthur, E., & Hancock, P.A. (2001). Navigation training in virtual environments. International Journal of Cognitive Ergonomics, 5(4), 387-400.
Abstract:
Virtual environments (VE) promise important opportunities as future interfaces to computational systems, especially where such technology can take advantage of strong human visuospatial capabilities. Although such synthetic environments often project homeomorphic physical representations of real-world layouts, it is not known how individuals develop representational models to match these environments. To evaluate this process, this experiment examined participant’s accuracy in reproducing triadic representations of objects, having learned them previously under 1 of 3 different conditions. The layout consisted of 9 common objects arranged on a flat plane. These objects could be viewed in a free VE, a static VE, or from the static view of a map. The first condition allowed active exploration of the environment while the latter two conditions allowed the participant only a passive opportunity to observe from a single viewpoint. Viewing conditions were a between-subject variable with seven participants randomly assigned to each condition. Performance was assessed by the response latency to judge the layout accuracy of three object triads from different rotated positions. Results showed a linear increase in response latency as the rotation angle increased in both the map and static VE conditions. In contrast, and like findings from real-world investigations, the virtual navigation condition did not show such an effect for orientation angle. These results suggest that the spatial knowledge acquisition from navigation in VEs can be similar to actual navigation when the viewing condition is unconstrained. One caveat being that, while performance was more robust once knowledge of the spatial layout was acquired, participants took significantly longer to learn the layout in the virtual navigation condition as compared with either the static VE or the map conditions. Given that such differentiated learning effects are due largely to limits to contemporary VE technology, our study confirms that VEs hold great promise for spatial navigational learning.
Your web browser doesn’t have a PDF plugin. Please download publication from the link above